The European regulatory landscape for medical devices has undergone considerable change over the past few years. Manufacturers continue to be challenged in the area of achieving (and understanding) compliance requirements, from pre-market to postmarket surveillence, under EU MDR and IVDR. “It’s practically impossible to keep up with the volume and velocity of scientific, analytical and performance data and meet the new obligations of risk management, performance evaluation and postmarket surveillance,” said Lana Feng, Ph.D., CEO and co-founder of Huma.AI, in a recent company press release. “Until recently, device and diagnostics manufacturers have been able to make do with a patchwork of tools and systems, and a largely manual and error-prone regulatory compliance process. MDR and IVDR have changed all that. Put simply, that status quo approach is suddenly obsolete.”
Huma.AI launched a postmarket surveillance solution that uses artificial intelligence and natural language processing (NLP) to help companies meet the stringent compliance requirements of EU MDR and IVDR. MedTech Intelligence recently sat down with Feng to discuss the hurdles device companies face in meeting these requirements and how leveraging machine learning and NLP can save companies time and improve the accuracy of the data curation process during the postmarket surveillance process.
MedTech Intelligence: What challenges do device manufacturers currently face in complying with the postmarket surveillance requirements of EU MDR and IVDR?
Lana Feng, Ph.D.: [EU MDR and IVDR present] a new kind of active surveillance of product performance and safety. There are three components in postmarket surveillance First, you have to actively monitor the performance of your products. What industry is doing now is mainly manually curating literature research, some from publication sources and others from private data sources. Second is the requirement that you have to do comprehensive reporting, [which includes culling] product recall or defects databases, and reviewing the private data such as complaints that you collected from customers. Lastly, you have to prove you are state of the art, meaning you are showing your products perform better than the competitors.
This is a very daunting task. If you take literature search as an example, you have to manually go through hundreds sometimes thousands of publications from keyword searches to curate it. The industry is trying to meet that challenge, and is relying on existing solutions, trying to hire as many business analysts and experts as they can. And some of the big manufacturers have hundreds of thousands of products. It just does not scale; it’s a huge problem.
MTI: Tell us about Huma.AI and how its solution can help manufacturers meet these stringent requirements.
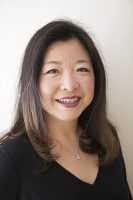
Feng: Great, let me tell you about Huma.AI and the genesis of the platform. The company was founded in 2018, based on my experience coming from precision medicine and life sciences where we have so much data—and 80% of it is unstructured, making it impossible to get insights out of data. What if we could make interrogating data as easy as using Google, turning domain experts into their own data scientists?
There are three key attributes of this platform that tie in nicely with postmarket surveillance. The first we call postmarketing intelligence in that it is self-service—literally just ask the questions and get the answers instantly. It’s giving the power of extracting insights and doing analysis into the hands of end users, the domain experts.
Second, [the platform] has an innovative way of using national language processing, which is an actively researched branch of machine learning. You can analyze across millions of publications. This becomes a solution that takes all the labor intensive and error prone manual work out of the process. Third, you can use natural language to connect different data silos to get that 360-degree comprehensive reporting in order to be compliant.
I also want to pull out a really important point: When people see AI and machine learning, their eyes glaze over, right? Our approach is very much the human element. H-U-M-A [Feng spells out the company name] .AI really is humans plus machines—humans always come first. We don’t call it artificial intelligence, we call it augmented intelligence, which is really important in life sciences because of our user experts. It’s very much augmenting what the experts are doing. In this case, taking the grunt work out of manual curation across many publications so that the experts can do the final QC and then incorporate it into their existing compliance, regulatory and post-market surveillance workflow. In addition, [the platform] it shows the logic so that the experts can inspect the logic behind the answer itself. They’re able to understand how the machine learning platform is coming up with the answer that it’s coming up with. And I think that’s super important.
MTI: In a resource-constrained environment, how can using a technology that leverages machine learning and natural language processing bridge the gap for companies?
Feng: The current status quo is human manual curation. So you hire more bodies, right? If let’s say I require one expert or data scientist to curate [data] for two or three products. That could take several weeks. What if you have 10 products? Or 50 products? You have to hire more people. There’s such a tight labor market, you just can’t hire that many people—it’s not sustainable. This is where we come in, again, to take the grunt work out of the equation.
I also want to say that our product has been validated through one of our customers to be 10 times more accurate, and that we’ve shortened the time from weeks to minutes. It’s a substantial improvement.
MTI: What’s on the horizon for Huma.AI?
Feng: The future is to build out a partnership with the industry to help them understand and reframe the challenges, and adopt new agile technologies, including machine learning and NLP to help them solve their complex regulatory, compliance and postmarket challenges. With that in mind, we are co-launching a partnership program that is designed for all stakeholders in the industry [to provide] greater access to resources, demos, understanding and interviews with experts, so that we can really dive into the challenges around identifying misuse and build a better product hand-in-hand with the industry. We want to bring life-saving products to market faster and safer, and help life sciences companies [do that].
It’s also about raising the level of discourse around how technologies can help people do their jobs better, not eliminate jobs. Huma.AI was created to augment humans, not to replace them.
Learn more about the the challenges, costs, and opportunities that EU MDR and IVDR have created, along with Huma.AI’s solution and vision for a New Era of Post-Market Intelligence in The Post-Market Gambit, After EU MDR and IVDR: A Systematic Compliance Framework for Driving MedTech Innovation.