Artificial intelligence, or AI, is transforming the medical device industry today. As medical devices continue to incorporate artificial intelligence to perform or support medical applications, new regulations require AI-driven medical devices to comply with state-of-the-art requirements and provide objective evidence for repeatability and reliability. AI has the potential to improve patient outcomes as well as the productivity and efficiency of healthcare delivery. It can also improve the day-to-day lives of healthcare providers by allowing them to spend more time caring for patients, hence improving staff morale and retention. It may even accelerate the development of life-saving therapies. Simultaneously, concerns have been expressed about the influence AI may have on patients, practitioners and health systems, as well as its potential risks; ethical arguments have erupted about how AI and the data that supports it should be utilized.
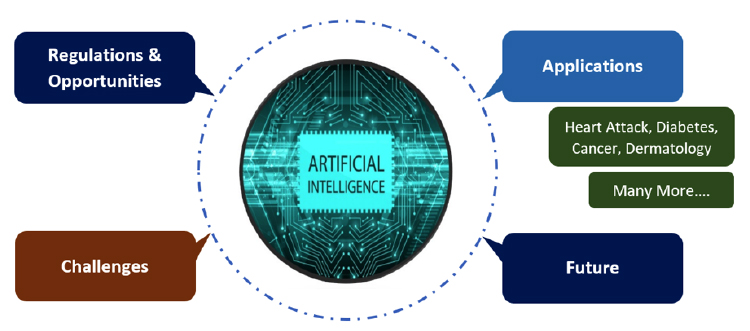
Leading researchers and clinical faculty members presented 12 technologies and areas of the healthcare industry that are most likely to see a major impact from artificial intelligence within the next decade at the 2018 World Medical Innovation Forum on artificial intelligence, hosted by Partners Healthcare.
AI in Medical Equipment and Healthcare
From real-time video from the interior of a refrigerator to automobiles that can identify when the driver is inattentive, smart gadgets are sweeping the consumer market. In the medical industry, smart gadgets are critical for monitoring patients in the ICU and elsewhere. Artificial intelligence can improve outcomes and minimize costs related to hospital-acquired diseases penalties by improving the capacity to predict deterioration, detect the development of sepsis, or detect the onset of complications.
Heart Attack
When a section of the heart muscle doesn’t get enough blood, it causes a heart attack, also known as a myocardial infarction. The longer the heart muscle goes without therapy to restore blood flow, the more damage it sustains. The most common cause of heart attack is coronary artery disease. A strong spasm, or abrupt contraction, of a coronary artery, which can block blood flow to the heart muscle, is a less common reason. Experts at the University of Oxford have used machine learning to create a “fingerprint,” or biomarker.
The fat radiomic profile reveals biological red flags in the blood arteries that feed blood to the heart, such as inflammation, scarring and vessel alterations, all of which are indicators of a potential heart attack. Another example is cardiovascular magnetic resonance (CMR): CMR is a scan that detects how much of a particular contrast agent the heart muscle picks up and evaluates blood flow to the heart; the stronger the blood flow, the less likely there will be blockages in the heart veins.
Diabetes
Uncontrolled diabetes causes diabetes mellitus, which can lead to multi-organ failure in individuals. As a result of improvements in machine learning and artificial intelligence, it is now possible to detect and diagnose diabetes in its early stages using an automated procedure that is more efficient than manual diagnosis. Image-based AI-assisted medical screening and diagnosis is presently in development. Diabetic retinopathy (DR), age-related macular degeneration, glaucoma, retinopathy of prematurity, age-related or congenital cataract, and retinal vein occlusion are among the disorders where this technique is now being applied in ophthalmology.
IDx-DR is the first AI algorithm authorized by the FDA for detecting DR in non-ophthalmic healthcare practitioners’ offices. The gadget is linked to a non-mydriatic retinal camera (Topcon’s TRC-NW400), which sends the pictures to a cloud-based server. Based on autonomous comparison with a huge collection of typical fundus photos, the server uses IDx-DR software and a “deep-learning” algorithm to discover retinal abnormalities compatible with DR. One of two outcomes is provided by the software: (1) Refer to an eyecare professional (ECP) if more than moderate DR is discovered; (2) If the results are negative for more than mild DR, rescreen in 12 months.
Cancer
One of the most promising methods of cancer treatment is immunotherapy. Patients may be able to beat tough tumors by attacking them with the body’s immune system. Machine learning algorithms and their ability to synthesize extremely complex data may open up new avenues for tailoring drugs to a person’s genetic composition.
Dermatology and Ophthalmology
Every year, the quality of cell phone cameras improves, and they can now create photos that can be analyzed by artificial intelligence systems. Two of the first specialties to gain from this trend are dermatology and ophthalmology. Researchers in the UK have even developed a gadget that analyzes photos of a child’s face to detect developmental difficulties. The approach may detect discrete elements including a child’s jawline, eye and nose placement, and other characteristics that could suggest a craniofacial aberration. The program can match everyday images to more than 90 illnesses, allowing doctors to make better-educated decisions.
Challenges
Electronic health records are a gold mine of patient data, but doctors and engineers have battled to gather and analyze it in a way that is accurate, quick and reliable.
Due to data quality and integrity difficulties, as well as a tangle of data formats, structured and unstructured inputs, and missing data, understanding how to engage in meaningful risk stratification, predictive analytics and clinical decision support has been particularly difficult. From cellphones with step trackers to wearables that can detect a pulse around the clock, a significant amount of health-related data is generated on the road. Collecting and analyzing this information, as well as complementing it with the information provided by patients via apps and other home monitoring devices, can provide a unique perspective on individual and population health. Artificial intelligence will play a key role in deriving relevant insights from this massive and diverse data set.
The difficulty of interoperability and integration is one of the main traits that divide academic research from practical AI applications. The majority of research focuses on creating AI models that function with carefully vetted sets of health data. Data is complex, scattered, and difficult to access in real life. The absence of a sufficient data architecture is often the biggest impediment to integrating AI into current applications.
Machine learning and artificial intelligence research require high-quality reporting. The danger of bias and the possible value of prediction models can only be accurately appraised if all features of a diagnosis or prognosis model are fully and clearly reported. Machine learning studies should aim to follow best practice recommendations like the Transparent Reporting of a Multivariable Prediction Model for Individual Prognosis or Diagnosis (TRIPOD), which is designed to help researchers report studies that develop, validate, or update a prediction model for diagnostic or prognostic purposes.
Conclusion
Artificial intelligence has great potential in medical science beyond what we can imagine, and the current applications are only the beginning. As briefly discussed, immunotherapy has significant promise in cancer treatment. As we know, cancer is a deadly disease that impacts vital parts of the body. Customizing diagnosis according to the patient’s genes is just outstanding. It is imminent that AI will help us in effectively diagnosing diseases, developing personalized medications for complex treatments, and much more.
In addition, many of the disease states discussed are a leading factor for a patient’s cause of death (i.e., heart attack). Not to forget the adverse events from diabetes are unimaginable—including cardiovascular complications, kidney damage, eye damage. Retinal image analysis also helps diabetic patients, as it aids the doctor with fundus image analysis, which can more swiftly help determine the next stages in a patient’s therapy. Doctors would be able to attend to more patients who require treatment. Emerging healthcare technologies focus on minimizing eye specialist visits, lowering total treatment costs, and increasing the number of patients seen by each practitioner. AI can assist the healthcare provider in reaching his or her objective more effectively.
Although this technology helps in the healthcare industry, it should not be used to replace a clinician time. Advances in AI are bringing with them new possibilities for running and grading algorithms. But as stated, this is just the beginning era of Artificial Intelligence-Machine Learning in medical science. The more we focus on the improvement of data quality and automation in the analysis of medical data, the more the algorithms can assist us in identifying useful patterns — patterns that can be used to make accurate, cost-effective judgments in complex procedures.