Why Should We Care About Digital Twins in Healthcare?
Have a look around and you see countless examples of devices monitoring and collecting data of our physiological state: Wearable smart watches and fitness trackers, medical imaging devices, digital health apps, thermometers etc. Similarly, these devices themselves are also producing large amounts of data about their current operational environment and condition. But how can we comprehend all these data and get meaningful insights from it? One possibility is to create a dynamic presentation of these data that is called a digital twin.
A digital twin is a virtual representation of a physical object or a system across its lifecycle. This means that the digital twin entails both up-to-date and historical data about the state of its real-world counterpart. Incorporating these dynamic data into the virtual representation for different medical applications enables proactive decision making, process optimization, and complete lifecycle management in healthcare.
Part 1: Digital Twin of a Human
When replicating a human or a patient, a digital twin is constructed using vital sign monitoring in combination with anatomical and physiological data. In the world of ubiquitous wearable devices and biomedical sensors, these data can come from multiple sources. For example, a smart watch can collect real-time information about the blood pressure, body temperature, pulse rate, sleep patterns and overall physical activity levels of the patient. Similarly, when the patient visits a clinic or a hospital, the virtual patient model can be updated with the data from the laboratory tests and diagnostic imaging studies conducted during the visit. Furthermore, genetic and behavioral data as well as social determinants of an individual could also be coded to the digital twin. When all these data are combined into a single virtual representation of a patient, a more complete picture of the medical history of the patient is available to support decision making.
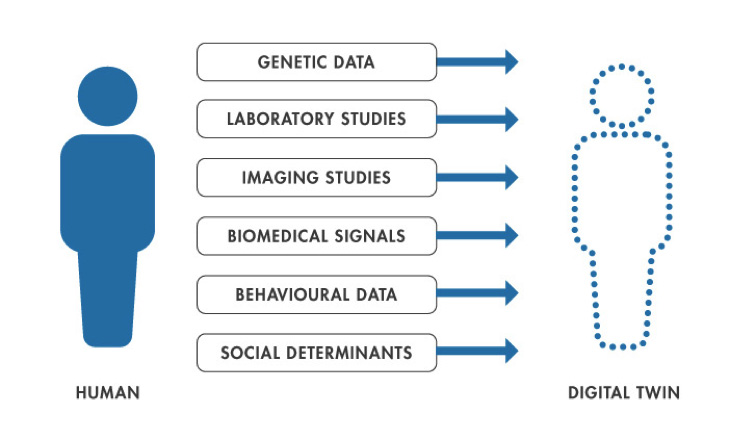
There are many potential applications for these virtual replicas of humans. For example, a digital twin of a patient together with AI models can be used in precision medicine to make proactive decisions about the right treatment options for the specific patient. A virtual human model could also be used in a simulation for testing novel medical therapies and drugs, as discussed by the FDA, that would otherwise be too risky or time-consuming if conducted on a real patient. For instance, a selection of chemotherapy drugs could be tested against the genetics and physiological processes of the patient in order to identify the best treatment response. Virtual models of individual organs can also be used in developing and testing new medical devices, such as heart models for designing pacemakers. These types of studies are commonly known as in silico medicine, which can be used to support or even potentially replace clinical trials in the future. From the perspective of a patient, a digital twin with vital sign monitoring allows proactive management of chronic diseases, fitness levels and overall health status. With all these data combined, people can make more informed decisions for personal health and thus achieve a healthier lifestyle.
Part 2: Digital Twin of a Medical Device
In the context of medical devices and technology, a digital twin is a virtual representation of a device in operation that captures its physical properties, environment and operational algorithms. A combination of different signals from embedded sensors can be used to gather information about the current health condition, configuration and maintenance history of the device. For example, the chiller in an MRI scanner—that is, the refrigeration unit to cool down the machine—can provide data about the historical operation temperatures of the imaging device, which could directly affect the remaining useful life of its parts. In addition, a large variety of other types of signals, such as vibrations, pressures, fluid levels and electrical voltages, as well as environmental parameters and device performance metrics can be collected to build an up-to-date virtual representation of a medical device.
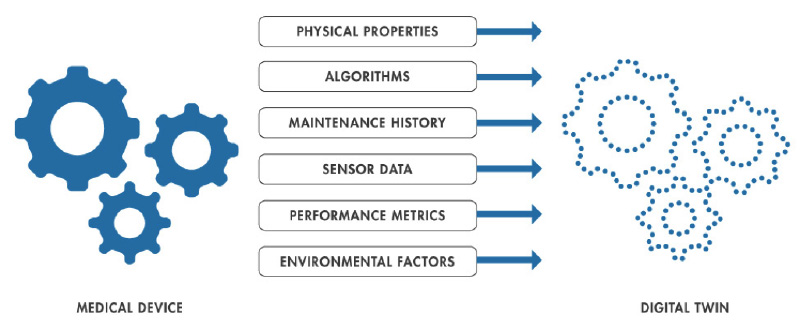
Medical devices are often safety-critical, and their failure could put patient lives at risk. Therefore, health monitoring and maintenance of the device are crucial. In a typical situation, the maintenance of a medical device is conducted either reactively or preventatively. In reactive maintenance the repair work is conducted only when the failure happens, which increases device downtime and might cause safety risks to a patient. In preventative maintenance the parts are changed proactively before the failure, when they still might have useful service life left. This approach is safer to the patient, but it increases costs due to more frequent maintenance. A digital twin combining the historical data of the device operation with machine learning models can be used to investigate the cause patterns that lead to failures before they arise. Also known as predictive maintenance, this approach maximizes the service life of device parts without compromising patient safety. Thus, a digital twin allows efficient and safe lifecycle management of a medical device.
Part 3: Digital Twin of a Hospital
Digital twins can also be thought of on a larger scale, where complete medical facilities or organizations can be simulated together with their dynamic operations and processes. An example of this would be a digital twin of a hospital, where departments and resources could be modeled to optimize their daily operations and improve safety. These facilities could be, for example, an intensive care unit, radiology department, patient waiting area or operating room. Simulations of these areas could incorporate a digital schematic of their floor designs, equipment locations, and logistics. A digital medical facility could also include operational data from hospital information systems such as staff schedules, administrative task and financial transactions. If all these data were combined in a virtual representation, the optimization of their operations could be achieved in a matter of days or weeks rather than after years of trial and error as in a physical environment.
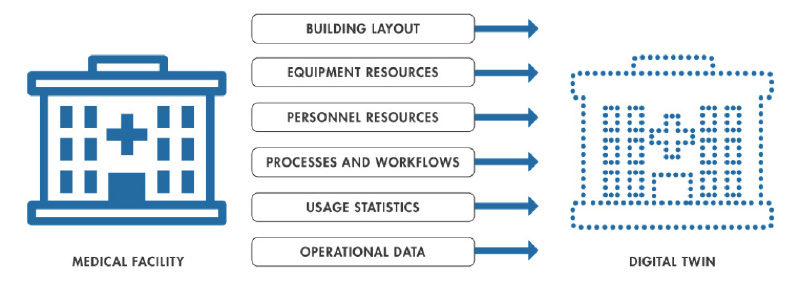
Virtual replicas of medical facilities could be used to increase equipment utilization rates and therefore their medical examination and operation capabilities. For instance, by visualizing the logistics of patient arrival all the way to finishing the examination and departure, the processes causing the biggest delays could be identified and optimized for faster patient turnaround times. This would result in shorter patient waiting durations and have a positive impact on customer and staff experience. Similarly, the staff schedules could be optimized according to the demand in clinical services. If the accident and emergency department sees the highest demand for emergency care at certain times during the week, the personnel resources could be dynamically adjusted to better account for the demand. A digital twin of a hospital would allow smarter resource allocation and increased operational flexibility without compromising clinical safety. All these aspects together contribute towards more patient-focused medical care and data-driven decision making.
Accelerating Digitalization in Healthcare
Digital twins are still a relatively new concept in healthcare, and the field is continuously evolving with more sophisticated virtual models enabled by better computational capabilities. Hospitals, clinics and medical device companies are also collecting increasingly more data to incorporate into these models, which makes them more accurate representations of their real-world counterparts. Digital twins are a step towards more personalized and value-based healthcare for patients. For healthcare providers and manufacturers, digital twins allow efficient process optimization and better product lifecycle management.