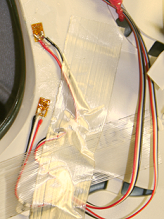
In this enlightened age of “intelligent engineering,” data and the interpretation of data have become critical to the success of a project. The development tools in our high-tech toolbox are always expanding and bringing new insight to increasingly complex systems. But without a detailed product specification roadmap, we fall into the trap of generating oceans of useless data. The design team of today needs to be keenly adept at gathering, filtering, and utilizing data for the best possible patient outcome as well as commercial success.
Engineers love data. We always want more high-speed cameras from more angles to record more prototype scenarios to verify more simulations. This brings us to one of a program manager’s greatest frustrations: usable data.
Whether you are designing an autonomous surgical robot, a patient-specific knee implant, or a paperweight for all the new regulations, volumes of data are useless without the proper specification framework to filter and process it. This is the role of the Product Requirement Document (PRD).
The PRD is designed to keep the development team on task and focused on specific product functionality. If the PRD is written at the end or evolves during the development program, truth-seeking engineers will generate ever increasing volumes of data. We do this to provide program decision makers a broad spectrum of options with varying degrees of feasibility. Working without a PRD is absolutely the slowest and most expensive way to develop a product. In a perfect world, every program would start with a detailed PRD backed by historical benchmarking data, but this is not an option for truly innovative technology looking for a production platform. Even without a PRD, the development team will still need critical, effective processes to reach definitive milestones and eventually a market-conquering product. Without hard stops during the process to critically analyze data, you will get stuck in never-ending loops of data gathering and end up creating more variables than answers.
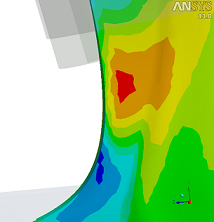
Because development resources are finite, brainstormed concepts need to be vetted quickly and either fail fast or prove promising and carried further. If hanging weights off a rapid prototype strapped to a car bumper gets you pertinent data in hours as opposed to machining prototypes and a detailed test fixture over four weeks, go with the car bumper. The most challenging aspect is to define the critical variables and harmonize them into a succinct goal. A great development team finds the facts in the figures and keeps the thought process moving forward quickly and efficiently.
One of the most powerful tools to reduce and accelerate prototyping loops is finite element analysis (FEA). FEA has made giant leaps forward in capability over the past 15 years. Large assemblies are now translated directly from any CAD environment, contact pairs are automatically assigned, and a mesh is created after the click of one button. It is the perfect tool to fail fast in both positive and negative ways. The results are shown plain as a rainbow on the computer screen, but are they fact? The quickest way to further a concept with FEA is through comparative analysis. If option A predicts X stress and option B is 0.5X stress with the exact same setup, you are moving in the right direction. The question then becomes are the physical stress levels at X or 10X.
There are many ways to validate FEA simulations in the real world. With the ever decreasing cost of 3D printers, you can be up and testing concepts in hours. The 3D printing materials will not be exactly representative of production materials (build layers, for example, are ideal crack slip planes), but remember that stress is force/area. Whether a part is printed or machined, both will have the same high-stress location when tested in identical setups just different ultimate loads and displacements. The issue arises when your prototype fails in ways you did not predict or expect. The failure load and location are in essence only one data point in a complex system. Would thinning out a section and increasing flexibility actually be more beneficial than thickening and stiffening a part? Does adding a gusset to support a corner relocate the high stresses to an even more critical location? To fully comprehend the behavior of a part or assembly, you need multiple data points within the system to trend or predict how your concept is performing as a whole.
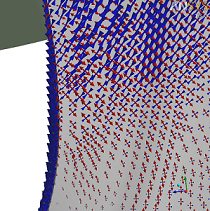
direction and magnitude of the three Principal Strains. Key for strain gage placement and alignment
Strain gages provide very enlightening data for validating FEA simulations. I have found over the years that many people either don’t understand or are afraid of using strain gages. The key is to confirm your gage application and data gathering process on something simple, like a bending beam, to start with. Perform the hand calculations, confirm the calculations with an FEA run, and match your physical gage readings to the predicted results. Once you validate your process, a whole new array of data gathering is available to physically map the performance of your concept. Strain gages have been immensely useful for sorting out FEA constraint issues and uncovering inappropriate setup assumptions. It is rare in the real world that anything is truly fully fixed. The development team will still need to iterate between the FEA setup/results and gage readings, but at least they will have a physical data path to correlation. It takes an experienced technical lead to understand when the analysis and physical testing are aligned well enough to predict the trend for your specific functional requirement. Strain gages are cheap, apply them liberally.
The desire to compress timeframes through the “intelligent engineering process” has become a reality across multiple industries. Development teams of today have the most incredible physical and virtual tools at their disposal, but they need to know how to most efficiently utilize them. Even without a PRD, data still needs to be gathered, sorted, and acted upon to develop and validate a concept. The right data in the right hands at the right time does make all the difference. Budgets, personnel, and time to market have all been significantly reduced, while at the same time product expectations have never been higher.