The FDA defines machine learning (ML) and artificial intelligence (AI) as follows2:
ML: “A system that has the capacity to learn based on training on a specific task by tracking performance measure(s).”
AI: “AI can use different techniques, such as ML, to produce intelligent behavior, including models based on statistical analysis of data, and expert systems that primarily rely on if-then statements.”
This article will examine some of the standard considerations that a device manufacturer must traverse to get their device to market. It then explores additional or alternative steps that manufacturers of AI-enabled devices must also consider throughout this process.
Traditional FDA Approval Pathways
There are three non-exempt FDA approval pathways: De Novo; Premarket Approval; and 510(k). When the manufacturers of typical non-AI-enabled devices are prepared to request FDA clearance for marketing, two major factors aid in determining which of these pathways is the most appropriate pipeline for their submission (exemptions are an irregular third factor that may also alter this decision). The first of these characteristics is the device’s designated FDA classification (see References below for FDA classification details). The second trait is an assessment of whether there is a recognized, substantially equivalent (SE) predicate device that has received FDA clearance for marketing.
The summary characteristics of each of the three main approval pathways include:
De Novo. The De Novo approval pathway is taken when there is no substantially equivalent predicate device. Approval is dependent upon demonstrable efficacy and safety while presenting minimal risk. Approved devices are designated as FDA Class I or Class II and can subsequently serve as predicate devices for future applicants with similar technology4.
Premarket Approval (PMA). The premarket approval pathway is the FDA’s most stringent approval pathway, intended for FDA class III devices. Because medical devices with a Class III designation carry a higher level of risk, the FDA requires higher quality, clearer evidence of benefit and safety for use in the intended population5.
510(k). By contrast, the 510(k) process is the least intensive of the three traditional approval pathways. For FDA class I, II, or III (only if exempted from PMA) devices, a manufacturer may submit an application that demonstrates substantial equivalence to a legally marketed predicate device. According to the FDA, substantial equivalence is met when statements either both condition 1 and 2a or 2b are met6:
- The new device has the same intended use as the predicate device
- Either one of the following:
- The new device has the same technological characteristics as the predicate device.
- The new device has different technological specifications that do not alter the efficacy or safety profile, and the submitted information in the FDA application provides demonstrable evidence of comparable safety and efficacy to the predicate device.
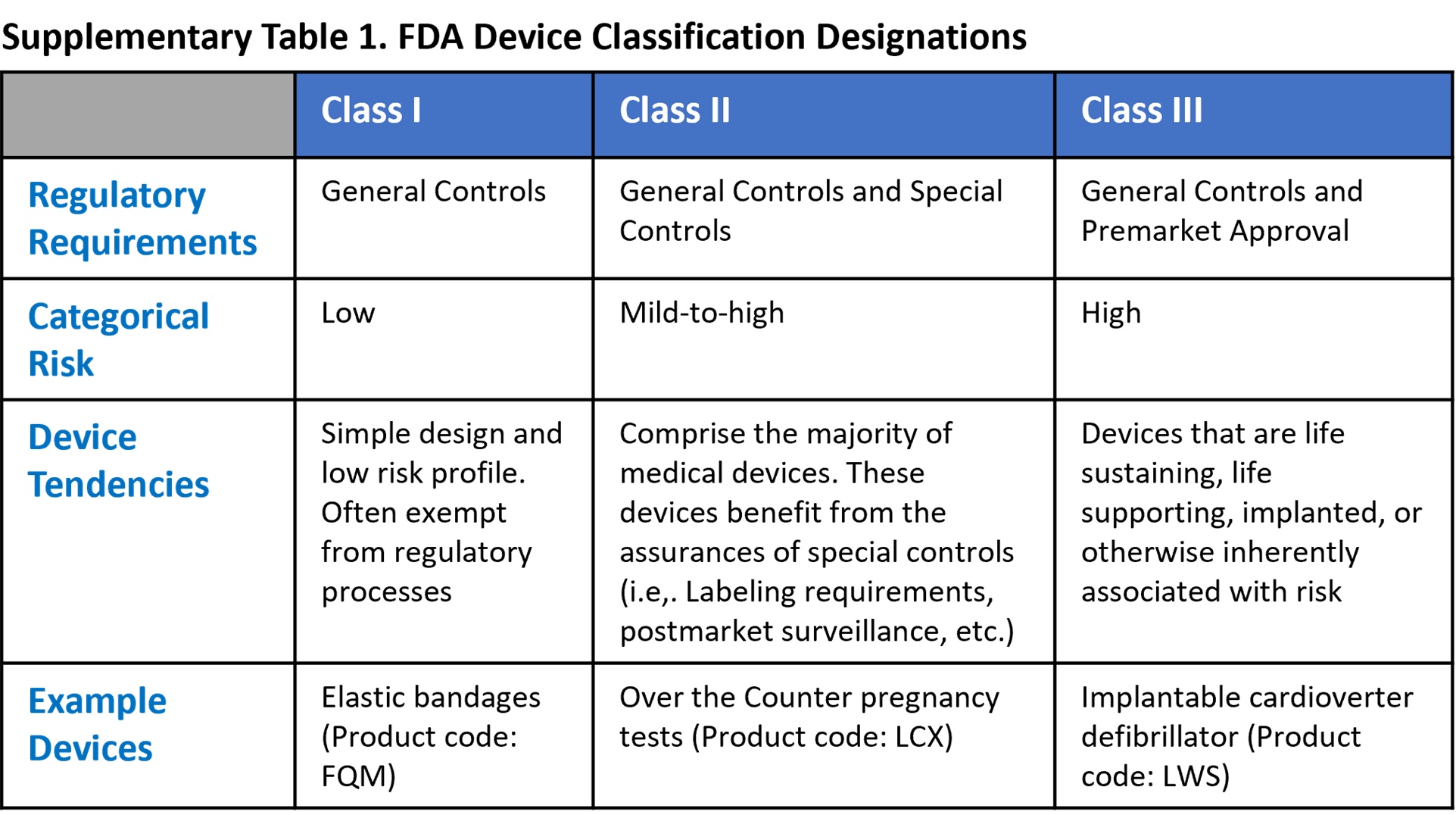
Unique Challenges and Special Considerations for AI/ML Devices
According to 2019 guidance from the FDA, AI/ML-enabled Software as a Medical Device (SaMD) manufacturers should use the same standards of device risk assessment and approximation of equivalent predicate devices as non-SaMD devices to determine which application pathway is the most appropriate for submission2.
However, there is a third component introduced in the prior 2017 edition of this guidance that can be an important supplement to consider in a device’s risk assessment. The 2017 guidance cites a risk assessment scale for SaMD created by the International Medical Device Regulators Forum (IMDRF). This risk assessment algorithm considers both the seriousness of the health condition as well as the significance of the device’s place in the ultimate healthcare decision/outcome to arrive at a final risk score ranging from I (lowest risk) to IV (highest risk)2. The IMDRF risk score can be a useful guide for drafting an intended use statement. In addition, in instances where the traditional product classification and substantial equivalence schema are unclear for an AI-enabled device, a manufacturer may choose to leverage an IMDRF risk score to aid their decision in selecting the approval pathway that is the most appropriate for their device’s FDA submission.
As of October 2023, the vast majority of SaMDs received approval via the 510k pathway. Of the 692 AI/ML-enabled devices reviewed, 96.5% (668) were cleared through the 510(k) pathway, 3% (21) were cleared through the De Novo pathway, and only 0.4% (3) were cleared via the PMA pathway. Furthermore, many of these AI-enabled devices were considered substantially equivalent to non-AI enabled predecessors1.
In the 2021 guideline for AI-enabled SaMD (Software as a Medical Device), the FDA mentions that steps for ensuring the approval of AI-enabled Software in a Medical Device (SiMD) would likely overlap with that of AI-enabled SaMD. However, no other FDA guidance yet exists for AI-enabled SiMD.
Adherence to Good Machine Learning Practice (GMLP). All manufacturers must follow the 2021 GMLP for Medical Device Development Guiding Principles issued jointly by the FDA, Health Canada, and the UK’s Medicines and Healthcare products Regulatory Agency8. This document outlines 10 characteristics to ensure an AI/ML algorithm is mitigating bias amplification, maintaining discrete training datasets, and transparent in decision-making.
Locked vs. Adaptive Algorithms (TPLC Regulatory Approach). Locked algorithms are those that are static over time. Conversely, adaptive algorithms are dynamic and continue to “learn” over time based on outcomes. As of their 2019 Framework for AI/ML-Based SaMD, only “locked” algorithms had received FDA approval1. For manufacturers of adaptive AI/ML algorithms, five guiding principles have been proposed as the guardrails for issuing predetermined change control plans to address the anticipated changes to the device’s algorithm throughout the total product lifecycle (TPLC) 8.
Looking to the Future
It is reasonable to expect that the FDA will develop additional guidance as it encounters new challenges in increasingly regulating AI-enabled devices. Until clear guidance exists (i.e., for applicants of AI/ML devices with adaptive algorithms), it is essential for manufacturers of these devices to communicate closely with the FDA throughout the entire application process so that obstacles can be navigated together.
AI technology represents a new and rapidly expanding category of medical devices that may hold the potential to improve healthcare by enhancing the effectiveness, efficiency, and equity of care delivery. However, this rapid growth has brought about unprecedented regulatory challenges. It is crucial for manufacturers of AI/ML-enabled devices who are seeking FDA approval to understand these nuances and special considerations involved with this category of devices to maximize the likelihood of approval and minimize time spent in the FDA review process. Adhering to FDA guidance and best practices and establishing transparent and frequent communication with the FDA are essential components of a successful application.
References
- US Food and Drug Administration (FDA). Artificial Intelligence and Machine Learning (AI/ML)-Enabled Medical Devices. Accessed 1/16/2024, https://www.fda.gov/medical-devices/software-medical-device-samd/artificial-intelligence-and-machine-learning-aiml-enabled-medical-devices
- US Food and Drug Administration (FDA). Proposed Regulatory Framework for Modifications to Artificial Intelligence/Machine Learning (AI/ML)-Based Software as a Medical Device (SaMD). Accessed 12/21/2023, https://www.fda.gov/media/122535/download?attachment
- US Food and Drug Administration (FDA). Artificial Intelligence/Machine Learning (AI/ML)-Based Software as a Medical Device (SaMD) Action Plan Accessed 12/21/2023, https://www.fda.gov/media/145022/download
- US Food and Drug Administration (FDA). De Novo Classification Request. Accessed 12/21/2023, https://www.fda.gov/medical-devices/premarket-submissions-selecting-and-preparing-correct-submission/de-novo-classification-request#De_Novo_Request_Decisions
- US Food and Drug Administration (FDA). Premarket Approval (PMA). Accessed 12/21/2023, https://www.fda.gov/medical-devices/premarket-submissions-selecting-and-preparing-correct-submission/premarket-approval-pma
- US Food and Drug Administration (FDA). Premarket Notification 510(k). Accessed 12/21/2023, https://www.fda.gov/medical-devices/premarket-submissions-selecting-and-preparing-correct-submission/premarket-notification-510k
- US Food and Drug Administration (FDA). 2017. Software as a Medical Device (SAMD): Clinical Evaluation – Guidance for Industry and Food and Drug Administration Staff (fda.gov) Accessed 01/16/2024.
- US Food and Drug Administration (FDA), Health Canada, UK’s Medicines and Healthcare Products Regulatory Agency (MHRA). Good Machine Learning Practice for Medical Device Development: Guiding Principles. Accessed 12/21/2023, https://www.fda.gov/media/153486/download